Unstructured Data Analysis
While there are tools available, unstructured data is still a lost puzzle piece searching for its perfect fit. As unstructured content grows, companies have adopted numerous storage solutions, making system consolidation difficult and universal oversight nearly impossible. Developers are currently still working on unstructured data analysis tools and creating best practices for their management and governance.
Within today’s organizations, both the volume of content and the number of applications deployed to manage that content are rapidly expanding.
These rising siloes are casting shadows over growing piles of unstructured data, obscuring sensitivity, security and ownership, and any other potential risks lurking within.
What is Unstructured Data?
Sometimes called unstructured information and classified as qualitative data, unstructured data is data that has no pre-defined data model or pattern and is, therefore, unorganized and not easily searchable by AI or machines. To add, unstructured data is data most often created by people, rather than by systems.
Unstructured Data Examples:
While structured data has a formal structure in place, unstructured data on the other hand, simply put, does not.
- Audio
- Text files
- PowerPoint presentations
- Social Media Data
- Video
- Mobile Activity
Unstructured Data VS Structured Data Example:
How Do You Analyze Unstructured Data?
Today’s global corporations have minimal insight into and control over one of their most valuable assets – their enterprise content. Content is everywhere: dispersed across cloud services, networks, local and remote offices, ECM platforms, and within business systems and applications.
Understanding the full scale of the content, its location, its value, and the business risk is an immense, difficult, and growing challenge, leaving organizations vulnerable to significant risk or lost opportunity.
If you cannot easily organize it, then how do organizations analyze unstructured data? The search-difficulty of unstructured data naturally makes its content analysis challenging.
While legacy approaches to managing content are constrained to a specific cloud service, on-premises storage system, or business application, there are technologies that integrate and unify all content sources.
DryvIQ provides a platform and suite of products that employ modern advances in artificial intelligence and machine learning technologies to seamlessly unify three core dimensions of enterprise data management, providing a single platform to discover, migrate and govern unstructured data across all connected content systems.
Unstructured Data and AI
The volume of information entering organizations, especially unstructured data, is accelerating at a staggering 50% per year. Manual methods of unstructured data analysis are costly and often fail due to fragmented storage systems and mismanaged solutions. Companies that cannot properly identify the information they possess run the continuous risk of security breaches and costly repercussions.
Machine Learning and Unstructured Data
Machine learning algorithms classify and label content by identifying sensitive, high-risk, obsolete, duplicate, and “dark” data.
Because machines can easily search for structured data, it’s expectedly easy for those machines to analyze that data. On the other hand, unstructured data requires additional processing since it is inherently difficult for machines to find.
Leveraging artificial intelligence processing, the DryvIQ platform continuously monitors for sensitive information or incorrectly applied labels that may expose your organization to loss of intellectual property, financial damage, or other vulnerabilities.
Act upon and safeguard at-risk content based on a series of configurable rules and policies. The DryvIQ platform will process the policy rules while notifying system administrators or other stakeholders in real-time.
Unstructured Data and Compliance
Companies must operate within ever-changing data compliance requirements and pressures such as GDPR, CCPA, and HIPAA. Relying upon the user to take appropriate actions to enforce governance policies and remain compliant, companies with mismanaged data simply cannot keep up.
With unstructured data growing at a rate of more than 50% per year, safeguarding that content can feel impossible. Relying on users and manual processes to ensure your content is properly managed is rife with opportunities for mistakes.
This lack of control opens organizations up to large fines, loss of sensitive data or intellectual property, operational inefficiency, or a negative impact on market and brand value.
This lack of control opens organizations up to large regulatory fines, substantial loss of sensitive data or intellectual property, unnecessary or redundant costs, operational inefficiency, and can negatively impact an organization’s overall market value.
Conclusion
Unstructured data analysis is no small hill to climb. Unstructured data is everywhere, growing, and difficult to find, making machines and AI necessary for organizations that want to fully leverage all their data to its full potential.
Thankfully, technology is getting smarter every day, making this a reality, and frankly, a necessity. With privacy and compliance mandates becoming stricter every day, it’s up to organizations to ensure their data is organized, managed, and compliant.
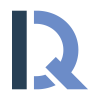