You might be surprised by how much data your business generates, even on a daily basis. Some of it—what’s called structured data—is relatively easy to deal with, as it is neatly formatted and organized in a database. It’s not hard to access this data, find exactly what you’re looking for, and trust that it’s accurate and up to date.
There’s also a vast category of data—unstructured data—that’s trickier to identify and properly handle. It refers to any data that’s not in database form, and can include things like documents and presentations, email and social media content, mobile data, multimedia, and so on. These types of data resist easy organization like a database would offer. Instead, organizations must deploy data classification methods that will collect, identify, manage, and store the wealth of data that’s generated in the course of doing business. Without sensitive data discovery tools and processes, this can be a tedious and error-prone endeavor.
This is especially important when you consider that sensitive data can reside in both structured and unstructured data sources. Without well-defined data classification levels and an effective governance framework, companies risk eroding customer trust (in the event of a data breach or unauthorized access), incurring steep compliance fines, and more.
What Is Data Classification?
In simple terms, data classification refers to the scanning and labeling of data, in order to categorize it based on predetermined criteria. This is commonly the data’s intended usage, sensitivity level, or the potential risk of misuse (from a cybersecurity perspective).
Why Is It Important to Classify Sensitive Data?
Without proper sensitive data classification, an organization opens itself up to many unnecessary risks—including, most notably, the loss of customer or client trust. Effective data classification and governance policies also help companies to avoid penalties levied for non-compliance with industry-specific regulatory bodies, such as:
- Sarbanes-Oxley Act (SOX), which helps to “protect investors from fraudulent financial reporting by corporations”
- Health Insurance Portability and Accountability Act (HIPAA), which implements “national standards to protect sensitive patient health information from being disclosed without the patient’s consent or knowledge”
- Payment Card Industry Data Security Standard (PCI DSS), which seeks “to enhance global payment account data security by developing standards and supporting services that drive education, awareness, and effective implementation by stakeholders”
- General Data Protection Regulation (GDPR), which requires organizations to “safeguard personal data and uphold the privacy rights of anyone in EU territory,” including “seven principles of data protection that must be implemented and eight privacy rights that must be facilitated.”
Ultimately, it is important to classify sensitive data in order for organizations to:
- Protect their intellectual property.
- Safeguard sensitive customer data.
- Keep data organized and easily-accessible.
- Prevent potentially catastrophic—and costly—data breaches.
What Are the 3 Main Types of Data Classification?
Generally, data classification is based on at least one of three systems, each of which we’ll take a deeper dive into:
- Data classification by context, content, or user
- Data classification by sensitivity level
- Data classification by intended use-cases or policies
Data Classification Based on Context, Content, or User
This type of data classification considers the context, content, or intended user of a particular piece or set of data.
- Content-based classification involves assessing files directly, and categorizing them based on either the type of content and its general sensitivity level.
- Context-based classification is useful when considering large sets of files from a single, identifiable source (as opposed to the specific content). Context-based classification accounts for the specific application or user that generated the data.
- User-based classification, a primarily manual process, sets classification and governance rules based on specific users, access levels, and risk factors.
Data Classification Based on Sensitivity Level
These data classification levels are based around specific user and use cases, as well as the potential risk/impact that could arise if it were to be compromised or destroyed.
- High-sensitivity data, the most serious classification level, applies to any data that could have a catastrophic impact on specific individuals or the organization as a whole. Financial records, personally identifiable information, and intellectual property are typically considered to be highly sensitive information.
- Medium-sensitivity data is meant for internal use only, and poses moderate risk to the organization if breached or otherwise compromised. While the risk attached to medium-sensitivity data wouldn’t rise to the level of catastrophe, it would still be a serious and potentially costly infraction.
- Low-sensitivity data is the type of content that is available to the general public—website content or press releases, for example. As such, it poses little threat when compared with high- and medium-sensitivity data.
Data Classification Based on Use-Case and Policy
This third and final data classification framework is highly adaptable to internal policies, as it is based around factors like who should be able to access the data and how long it should be retained by the organization. It essentially combines elements of the two frameworks described above. For this framework, there are four categories, listed below in order of increasing sensitivity:
- Public data is accessible to everyone—in other words, it is for both internal and external use. For this reason, public information (like website content) is typically categorized as low-sensitivity data.
- Internal-only data is meant to be accessible to everyone within an organization. This category includes items like internal communication or business planning. Based on this, internal data would generally classify as medium-sensitivity data.
- Confidential data requires special authorization and clearance before it can be accessed, as it can include medium- and high-sensitivity data, such as payment card details, Social Security numbers, and HIPAA-protected information.
- Restricted data is the strictest classification, with potential penalties for unauthorized access or use including substantial fines and potential criminal charges. Organizations with a large amount of proprietary intellectual property tend to set rigorous standards for restricted data governance.
Why Is a Data Classification Policy Important?
When you consider the different types of data classification and the potential risks associated with poor sensitive data collection, handling, and governance, the importance of a detailed data classification policy becomes clear. As you can imagine, depending on the business type or industry, there will be a number of factors to consider when classifying sensitive data. This way, specific rules and workflows can be developed in order to make sensitive data classification a repeatable and reliable process.
An organization’s data classification policy might include factors like:
- Who, within the company, is responsible for data collection and handling
- Standardized tasks and procedures for collecting, processing, organizing new data
- Rules for who can/can’t access specific data
- Ongoing data security best practices and systems
- Compliance with industry-related standards and regulatory bodies and requirements
Discover and Classify Your Organization’s Sensitive Data with DryvIQ
If you work with data—this almost certainly includes you—then you know how important it is to classify sensitive data. Dealing with structured data—the kind of neat and tidy data held with an organized and easily-accessible database—is one thing, but unstructured data presents a number of unique challenges. One of the biggest difficulties with unstructured data is discovering what you have in the first place, leading to questions like:
- How much data are we collecting?
- Where does our data reside?
- How sensitive is it?
- How is it being protected?
- Who has access to this data?
Fortunately, enterprise data management tools like DryvIQ can have your back. Our platform helps companies manage the challenges of unstructured data, mitigating costly risks. With DryvIQ’s unstructured data discovery and classification tool, companies can:
- Maintain compliance data.
- Lower the risk of data loss.
- Lower the risk of regulatory fees.
- Reduce operational costs.
- Improve the IT team’s ability to do their job successfully.
Schedule a demo to see the DryvIQ platform in action today!
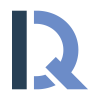