Since the beginning of business, there has been data. Sales data, customer data, expense data… the list goes on and on. As society and industries have evolved over time, so too has the data they rely on. In this day and age, you’d be hard pressed to find a website that doesn’t mention something about “data-driven this” and “actionable data that.” The fact of the matter is, businesses run on data, and they produce tons of it daily.
With so much data living in the ether, it tends to fall into one of two categories – structured or unstructured. Structured data can be collected and organized neatly in spreadsheets and databases. This makes it easy to access, understand, and find exactly what you are looking for. On the other hand, unstructured data can be quite the opposite. Unstructured data is more difficult to identify and handle due to the fact that it’s not in database form. Oftentimes, unstructured data is hard to find, secure and organize and therefore can be seemingly impossible to navigate. Sensitive data discovery changes all of that.
Sensitive data discovery takes unstructured data and helps classify it into organized categories and manageable clusters. In the following blog, we are going to look at data classification levels, and how unstructured data can be stored in organized, accessible, and user-friendly formats.
What Is Data Classification?
Data classification is based on the process of organizing and storing data in an effort to categorize it based on certain criteria. The point of data classification is to create relevant categories of data to make it easier to locate and retrieve data.
What Is the Benefit of Classifying Data?
There are several benefits of data classification. They include the ability to:
- Support regulatory compliance for sensitive data. Governing institutions like Health Insurance Portability and Accountability Act (HIPAA) and General Data Protection Regulation (GDPR), among others, require certain standards, protection, and classification of data. Additionally, classifying data also makes it easier to adapt to any new or revised regulations or rulings.
- Lower the risk of data loss. By identifying and organizing private and sensitive data, it is easier to protect it properly.
- Reduce operational costs. Having a better understanding and management system of your unstructured data can save money in storage costs as well as data retrieval.
- Allow employees to do their jobs with ease. Instead of spending hours mining through unorganized data, your team can focus on managing and optimizing data operations instead.
What Are the 4 Types of Data Classification?
Generally speaking, there are 3 main types of data classification that people will talk about which are: context, content, and user. In addition however, at DryvIQ, we believe that a fourth classification level, risk, should also be taken into account. Let’s take a look at these 4 data classification examples.
Context-Based Classification
Context-based classification involves looking at applications, locations, creators, and other variables as indicators of sensitive information and unstructured data. In other words, it is useful when considering large sets of data from one single identifiable source.
Content-Based Classification
Content-based classification evaluates data by assessing the files directly and placing them in categories based on the type of content and/or its sensitivity level such as high, medium, or low.
User-Based Classification
User-based classification relies heavily on a manual end-user selection of each file. Simply put, user-based classification involves a knowledgeable user making a judgment about how sensitive data is and how best to classify files. It can also refer to rules based on specific users and their access levels and risk factors. This can take a lot of time however, unless automated with data discovery software, like DryvIQ.
Risk-Based Classification
In addition to the traditional classification methods listed above, it also makes sense for organizations to consider the risk levels associated with certain data. Another way to think about risk is to think about sensitivity. For example, highly sensitive data is high-risk data. Generally speaking, risk level breaks down into three parts: low, medium, and high.
- Low-Risk Data is content that is typically available to the public and is easy to recover if lost. Because it is publicly available, there is little to no risk associated with it. This might include data from website content or press releases for example.
- Medium-Risk Data is meant for internal use only, as opposed to being openly accessible to the public. Medium-sensitivity data might include things such as proprietary operating systems or company budgets. Losing or leaking these documents wouldn’t necessarily spell catastrophe, but it would still be considered a less than ideal scenario.
- High-Risk Data is the most serious classification level and refers to anything remotely crucial or sensitive to operational security. Confidential, sensitive, and operationally-necessary data all falls under this umbrella. Data that would be incredibly difficult to recover would also qualify as highly-sensitive. If it is leaked or lost it could be disastrous for the company.
Discover and Classify Your Data With DryvIQ
Everyone works with data, probably more than you might consciously realize. That’s why it’s important to secure your data and classify it properly. The reality is that dealing with structured data can be straightforward enough, but organizing unstructured data might seem impossible. At DryvIQ we not only make it possible, we take the manual effort out of the equation by using proprietary artificial intelligence (AI) models to discover and classify unstructured data at scale. Schedule a demo today to see DryvIQ’s unstructured data discovery in action.
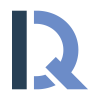