Sensitive data discovery involves locating data that requires protection so you can ensure it is secured against compromising situations. Well-known examples include passwords and social security numbers, but the scope of sensitive data is much broader than those items. So, before we jump into sensitive data discovery tools, let’s backtrack a bit and define what sensitive information is.
What Is Sensitive Information?
Sensitive information is structured or unstructured data that could be used to cause harm or loss of advantage if it ends up in the wrong hands, like trade secrets or customer lists and databases. There are many types of sensitive data, such as:
- Personal Identifiable Information (PII) – PII includes information that identifies an individual like their name, address, social security number, phone number, etc.
- Nonpublic Personal Information (NPI) – NPI includes personally identifiable financial information that is not publicly available, like bank account numbers.
- Material Non-Public Information (MNPI) – MNPI includes company data that has not been released to the public that could also influence share price, like upcoming acquisitions or other influential corporate actions.
- Protected Health Information (PHI) – PHI is similar to PII, but specifically covers identifiable health information, like information put into your medical records by health care providers or billing information between you and your physician.
- Confidential, Regulated, and High-Risk Business Information – This category overlaps with the other categories, as it includes similar data, but it’s data that is specifically sensitive to organizations, such as patents or financial information.
There are other types of sensitive data, but these well-known categories give a good idea of what sensitive data is. Sensitive data can exist in structured and unstructured formats, but unstructured, sensitive data is generally more at risk.
What Is An Unstructured Data Example?
A few examples of unstructured data include:
- Word processor documents
- Photos
- Videos
- Call transcripts
- Audio files
- PDFs
The full list of unstructured data sources would be much lengthier, but these examples are some of the most common. Think of unstructured data as having more qualitative characteristics than quantitative. It’s not organized based on a predetermined model, which makes it difficult to search and analyze.
Structured data, on the other hand, is formatted so that algorithms and data mining tools can access and analyze it. This makes finding sensitive, structured data significantly more straightforward than identifying sensitive, unstructured data.
To illustrate, let’s use a hypothetical scenario with structured, unstructured, and sensitive data. Sheila, a customer success team member at a SaaS (software as a service) company, is training a new hire to the team, Todd. Todd doesn’t have access to the customer database yet, where the company keeps all their customer information in a structured format. For training purposes, Sheila takes a screenshot of a portion of the database and emails it to Todd. The screenshot contains Personal Identifiable Information (PII), like customer addresses, social security numbers, phone numbers, etc.
That screenshot within the email is a perfect example of unstructured data containing sensitive information. And unfortunately, when Sheila emails the screenshot, that sensitive information is no longer protected by the fortress of firewalls and multiple security measures that safeguard the customer database. Hacking into one email account to steal that information would be significantly easier than breaking into the company’s network.
How Do You Find Sensitive Data to Ensure It Is Properly Protected?
This is where sensitive data discovery and classification come into play. If you can’t locate sensitive information within unstructured data, you can’t secure it. This leaves your company vulnerable to numerous threats, like identity theft and fraud, which in turn can lead to regulatory consequences, lost customer trust, and even lawsuits.
Fortunately, through unstructured data discovery, you can avoid these problems.
What Is Unstructured Data Discovery?
Unstructured data discovery is the process of analyzing an entity’s known and unknown data, classifying it, and then cataloging it. While your organization undoubtedly aims to protect sensitive data in all forms, it can be easy to miss data when in an unstructured format, like that emailed screenshot of sensitive client information in the above example.
How do you conduct data discovery, then classify and catalog the data you find? Before you can begin this process, you should be well-versed in data protection regulations and machine learning. This is because:
- Data regulations are complicated and require an in-depth, comprehensive understanding to ensure proper compliance.
- Manually conducting unstructured data discovery without machine learning could take years instead of hours.
If you’re not an expert in data protection regulations or machine learning, not to worry, as many organizations use an unstructured data discovery platform like DryvIQ. At DryvIQ, we use artificial intelligence (A.I.) and machine learning models to locate and classify unstructured data for our customers. This means our customers get:
- Deep insights into their content repositories
- Exposed vulnerabilities and potential financial impact
- Automated actions to mitigate current and future unprotected sensitive data risks
Wondering about the potential risks that may exist in your unstructured data? Learn more about how DryvIQ can help you with unstructured data discovery here. If you’re ready to see our platform in action, schedule a demo with us today!
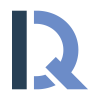