Following the explosion of generative AI (GenAI), 2023 was all about experimentation. Enterprises explored the possibilities of innovative technologies like ChatGPT, Bard and others. However, as we progress into 2024, the focus has shifted from experimentation with standalone tools to driving real business value with enterprise-level AI integrations like Microsoft Copilot.
Business leaders are eager to deploy GenAI across various use cases to optimize operations, improve customer experiences and automate tasks. However, deploying these models without strategic consideration can cause skewed outcomes, business risk and eroded stakeholder confidence.
Beyond strategy, a critical question looms: Do you trust your data? Trustworthy data is essential for unlocking innovation and driving a competitive advantage with GenAI. The quality of insight from GenAI is directly proportional to the quality of the data it receives; reliability, accessibility and accuracy are imperative to unleash AI’s full potential. From disparate repositories to complex data ecosystems, the road to actionable intelligence is often marred by inconsistencies, silos and uncertainties.
To generate business value from GenAI, strategic deployment is paramount, requiring unwavering trust in an organization’s data. Gaining that trust requires rethinking how we deploy GenAI and how we manage the hygiene, quality and security of our unstructured data.
Embrace The AI Journey With Confidence, Conviction And Clarity
Leaders should thoroughly assess the value of any GenAI initiative before scaling it. Recent digital transformation hype serves as a cautionary tale: A 2023 study by KPMG found that 51% of U.S. companies’ digital transformation investments haven’t caused a performance increase.
To protect your GenAI journey from a similar fate, start with high-value initiatives. Not all productivity or business value unlocked is equal; improving the productivity of an IT help desk employee by 10% may not have the same impact as that of an enterprise account executive. According to a recent McKinsey report, about 75% of the total value from GenAI use cases will stem from just four of 16 business functions: customer operations, sales and marketing, software engineering and research and development.
Conducting a value assessment and targeting high-impact GenAI use cases before enterprise rollout will boost confidence in the technology’s ROI potential.
Data Quality And Security Have Always Been An Issue—GenAI Has Exposed It Further
The effectiveness of GenAI hinges on data quality and security, particularly with unstructured data, which constitutes 90% of all enterprise data. The nature of unstructured data—the volume, variety and continuous creation, updating and sharing of documents by knowledge workers—makes ensuring quality and security challenging.
Data quality isn’t a new issue; hygiene and security concerns have plagued the governance, risk and compliance world for years. The problem now is how easily GenAI can expose these vulnerabilities.
Consider inadequate user permissions. If an employee has access to a document containing sensitive information, the risk is low if the employee is unaware the document exists. However, if a large language model (LLM) can use all the employee’s accessible data, it could reveal the information through one of its outputs. The risk is magnified when this unauthorized access involves employee or customer PII, intellectual property or other company confidential information.
Beyond increasing risk, data hygiene impacts the quality of GenAI outputs. Duplicate documents cause hallucinations and false relevancy in AI outputs, diminishing the models’ reliability. Additionally, “relevancy” varies by use case, and stale data in training datasets results in inaccuracies and outdated outcomes.
Insufficient insight into the cleanliness and security of unstructured data involved in AI training can erode trust in the accuracy and quality of the outputs while increasing the risk of exposing sensitive information—negating the value of deploying the technology in the first place.
So, how do we cultivate trust in our data?
Increase Confidence By Making Unstructured Data Accessible, Analyzable And Actionable
CIOs have long stressed the need to bring structure to their unstructured data. The solution to improving data quality and unlocking the value of this trove of information for GenAI use cases lies in making unstructured data accessible, analyzable and actionable.
Not all enterprise data is appropriate for use in the GenAI process. Identifying and organizing relevant, up-to-date information within unstructured is challenging but crucial for GenAI processes. Curating use case-specific document sets from disparate repositories, continuously updating them and purging stale data and excluding duplicates can reduce noise and biases, accelerate LLM training and enhance relevance and accuracy.
Carefully managing access rights to prevent unauthorized exposure of sensitive information is also critical. This includes managing not just what users can access but also the data that LLMs are trained on.
Investing in scalable discovery, classification and management of unstructured data is fundamental to cleansing, cataloging and readying your enterprise data for GenAI. Without mechanisms to automatically govern the accuracy and relevance of your data while securing sensitive information, confidence in the integrity of your training data (and the value GenAI can provide) will be compromised.
Steps To Power Your GenAI Journey With Trusted Data
Trust is crucial for AI success. A 2023 report by Cisco revealed that 62% of consumers worry about AI use, and 60% have lost trust in organizations due to AI practices.
Before getting swept into the GenAI hype, prioritize these steps to power your GenAI journey with trusted data.
- Focus GenAI Initiatives On Value: Invest first in high-value projects aligned with core objectives.
- Build Data Trust: Ensure the accuracy, relevance and security of your data with discovery and classification before using it for AI training.
- Test Thoroughly: Conduct small-scale tests to identify and address risks before enterprise-wide rollout.
Businesses embarking on GenAI initiatives need relevant, trusted data to avoid yet another futile digital transformation endeavor. As leaders, we have avoided addressing unstructured data challenges for too long. Gaining control and improving our data management practice enhances the quality and security of our enterprise data, giving us the ability to unleash GenAI’s full potential and generate immense value.
This article was originally published with Forbes Tech Councils.
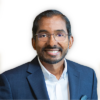