Generative AI is transforming how enterprises approach innovation and decision-making. But like any groundbreaking technology, its success depends on a solid foundation: data. And not just any data—clean, well-organized, and secure data.
Unfortunately, many businesses underestimate how poor data quality can derail their AI efforts. From security vulnerabilities to inaccurate outputs, bad data isn’t just a technical issue; it’s a business risk with serious consequences.
In this article, we’ll explore why data quality is critical for successful GenAI initiatives and share practical steps to ensure your data is AI-ready.
The Data Quality Problem
Bad data isn’t just an inefficiency—it’s a liability. According to Gartner, poor data quality costs businesses $12.9 million annually. For enterprises implementing GenAI, the costs can quickly multiply due to the reliance on accurate data for training models and generating predictions.
Beyond financial costs, poor-quality data can lead to serious risks and trust issues:
- Security Risks: GenAI can expose sensitive data when access controls are insufficient. For instance, if a large language model has access to unsecured documents containing customer PII or employee details, this information could inadvertently appear in its outputs. The potential fallout? Data breaches, compliance violations, and shattered reputations.
- Quality Failures: Duplicate, redundant, or outdated documents in datasets can result in errors like hallucinations and irrelevant recommendations. These inaccuracies diminish the reliability and usefulness of AI-driven insights.
For enterprises aspiring to stay competitive and secure in their data initiatives, investing in better data hygiene isn’t optional—it’s essential.
How to Build Confidence in Your Unstructured Data
Clean, organized, and secure data is the lifeblood of effective GenAI initiatives. To ensure your data is business-ready for AI, ask four critical questions:
1. Is your data relevant to the use case?
Have outdated or redundant files been removed? Only the most relevant data should remain accessible for AI models, eliminating unnecessary distractions.
2. Is your data organized for training?
Proper classification and organization simplify AI model training. If your data lacks clarity, model training will be inefficient, and outcomes will lack meaning.
3. Is your data cleansed to meet quality standards?
Measures like encryption, redaction, and anonymization ensure data aligns with privacy regulations and business rules.
4. Is your data secure?
Robust access controls protect sensitive information from exposure during model training and deployment. By limiting data permissions for both humans and AI models (like LLMs), risk is significantly reduced.
Answering these questions is the first step toward creating high-quality datasets tailored to your unique AI objectives.
Supercharge AI Success by Making Data Accessible, Analyzable, and Actionable
The solution to improving data quality and unlocking the value of this trove of information for GenAI adoption and effectiveness lies in making knowledge worker content, continuously accessible, analyzable, and actionable.
Not all enterprise data is appropriate for GenAI. Identifying and organizing relevant, up-to-date information is challenging but crucial for GenAI initiatives. Curating use case-specific document sets from disparate repositories that are continuously updated to purge stale data and exclude duplicates will reduce noise and biases, accelerate LLM training, and enhance relevance and accuracy. These data catalogs also serve as a “Bill of Materials,” providing oversight of which documents were used in training.
Carefully managing access rights to prevent exposure of sensitive information is also critical. This includes managing not just what users can access but also the data that LLMs are trained on.
Investing in scalable and continuous data scanning, cataloging, and automatic management of enterprise data is fundamental to ensuring it’s always business-ready for GenAI.
The Competitive Edge of High-Quality Data
Modern enterprises can’t afford to overlook the strategic importance of robust data practices. A Deloitte survey of 1,600 global leaders revealed that enterprises prioritizing data analytics and AI derive significant value, boosting efficiency and customer loyalty.
High-quality data empowers enterprises to:
- Reduce operational inefficiencies
- Enhance decision-making with accurate insights
- Strengthen customer relationships with personalized engagements
By fixing the challenges posed by unstructured data, specifically knowledge worker content, organizations can position themselves to achieve stronger AI outcomes.
The success of your Generative AI initiative hinges on the quality of your data. By prioritizing clean, secure, and well-organized datasets, you can set the foundation for AI models that deliver accurate, actionable insights while protecting sensitive information.
It’s time to take control of your unstructured data and position your enterprise for long-term success. Start by assessing your data readiness, addressing gaps in quality and security, and adopting scalable tools that simplify ongoing unstructured data management. Ready to take the first step? Contact us today.
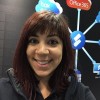