After years of experimentation, AI adoption is at the forefront of enterprise strategies in 2025. According to a recent market study on Enterprise Data Transformation by the Intelligent Enterprise Leaders Alliance:
- 55% of companies are increasing their budgets for AI readiness this year.
- 85% are doubling down on foundational investments like data governance and management, while 60% are also prioritizing data security.
- 75%—a significant majority—say aligning data initiatives with overall business goals is a top priority.
While we’ve reached a consensus that GenAI is the future and that data quality is critical for success, the challenge of how to obtain the right level of data quality remains. At the core of this challenge is the fact that data quality is not universal across all AI use cases; the data that is relevant to one initiative may not be to another. Just as poor data quality can sabotage even the broadest of GenAI initiatives, a one-size-fits-all approach to data quality can be just as crippling, especially when AI initiatives are linked to specific business objectives with high-stakes outcomes.
To drive true business impact with GenAI, data quality strategies must be use-case-specific and aligned with business goals.
AI Readiness vs. Reality
While executives push to increase AI adoption and dream of a seamless path to AI readiness, the reality is far more complex. According to a recent report from Accenture, 47% of CXOs said data readiness is the top challenge for generative AI. With the rush to deploy AI technologies at scale, IT teams are now faced with the challenge of preparing vast, disconnected and unorganized data stores, including the daunting task of dealing with unstructured data repositories—all the digital workplace and productivity applications employees use daily.
One of the biggest hurdles lies in managing the knowledge worker content generated, updated and shared by employees daily. This content holds the most relevant information for any data-driven use case and immense, untapped potential for generative AI initiatives. But it’s notoriously difficult to organize, classify and prepare for analysis, as this value typically lives within the contents of the documents themselves rather than within the file attributes.
To further complicate matters, many organizations invest in preparing their data without a clear understanding of what they’re preparing it for. Is the AI initiative focused on improving customer experiences? Is the objective to automate administrative tasks to improve operational efficiency? Without a vision for the application of generative AI, data preparation efforts risk becoming unfocused and disjointed.
Why Data Quality Is Not One-Size-Fits-All
Applying uniform rules across all data can lead to inefficiencies and missed opportunities. Different GenAI use cases have different requirements, meaning datasets must be tailored to serve specific objectives. For example, the data required to train a chatbot that improves employee digital experiences vastly differs from the data used to automate internal operations—and even department-specific internal initiatives may have differing data requirements. Taking a use-case-driven approach confirms that data relevance, organization, cleanliness and security (ROCS) are addressed in alignment with strategic goals.
The 4 Foundational ROCS Of A Data Quality Strategy
Relevant, clean, organized and secure data is the lifeblood of effective GenAI initiatives. To ensure data is business-ready for strategic AI initiatives, it’s essential that those tasked with data preparation ask four critical questions, following what we coined the ROCS Framework:
Relevance: Has redundant, obsolete or trivial content been archived to ensure the most relevant data is accessible for the specific AI use case?
Organization: Is the data organized and classified in a way that accelerates model training, or does it lack the categorization and clarity needed to drive meaningful outcomes?
Cleanliness: Has appropriate redaction, encryption or anonymization been applied to ensure the privacy and integrity of the data according to business rules?
Security: Are robust governance and access controls in place to protect sensitive information during the training and deployment of AI models?
Methodology For Strategically Preparing Data for GenAI
Step 1: Conduct A Values Assessment & Align AI Use Cases
What are you trying to achieve through AI? Conduct a values assessment and target high-impact GenAI use cases. By prioritizing use cases that align with your overall business objectives, your organization has a foundation upon which data can be prepared strategically to deliver the strongest outcomes.
Step 2: Perform An Inventory Scan
Audit your unstructured data repositories to catalog knowledge worker content across the entire organization. Understand the data at your disposal to determine what’s usable and how it can support the initiatives that surfaced from the values assessment.
Step 3: Apply The ROCS Framework To Enhance Data Quality
Curate high-quality, up-to-date datasets tailored to your identified use cases. Automated data management can enhance data relevance and security more efficiently while maintaining rigor in cleanliness and organization.
Taking this strategic, use case-specific approach to building your data quality strategy drives better outcomes for generative AI models, reduces waste by eliminating unnecessary data preparation and enhances collaboration between IT teams and organizational leaders.
Those Who Methodically Attack Their Data Today Will Lead In AI Tomorrow
As the potential (and demand) for AI to reshape revenue and operational efficiencies grows, a one-size-fits-all approach to data won’t cut it. AI readiness is not about having all the data—it’s about having the right data tailored to the right objectives. While this might seem like an unconquerable challenge, we are working with organizations that are methodically attacking their data and driving better outcomes from their AI initiatives—and those who embrace this data quality strategy will not only stay ahead but set the pace for the AI-driven future.
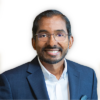